PDB
COMMUNITY FOCUS
Gregory Warren, Ph.D. OpenEye Scientific Software, Inc.
Gregory Warren, Ph. D.
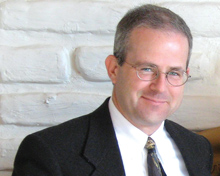
Gregory Warren did his graduate studies at the Massachusetts Institute of Technology with Gregory Petsko and Robert Griffin, and his post-doctoral work with Axel Brünger at Yale as part of the CNS development team. He spent eight years at GlaxoSmithKline as a computational chemist/molecule modeler
supporting drug discovery. In 2006, he
joined OpenEye Scientific Software, Inc. (www.eyesopen.com) as a Senior Applications Scientist where his responsibilities include support of AFITT OpenEye’s X-ray crystallography structure determination application. OpenEye develops large-scale molecular modeling applications and toolkits (programming libraries suitable for custom development). Primarily geared towards drug discovery and design, areas of application include structure generation, docking, shape comparison, charge/electrostatics, chemical informatics and visualization. The software is designed for scientific rigor, as well as speed, scalability and
platform independence.
Dr. Warren was recently one of the presenters at the Crystallography for Modelers course.
Q: What do you see as the balance between experimental/wet science and computational science in industry? How about in drug development?
A: Since my experience is in drug discovery and development, I can only comment on that particular aspect of industry. Unfortunately, computational science has been negligent. We rarely know or spend time determining the errors in or the true predictability of the methods we use. As a result, the perception is that experimental/wet science is the gold standard, and computational science is only useful when experiments are too expensive or when the number of experiments is too large. A model cannot be better than the data upon which it is built, so computation science will never “beat” experimental science. We can generate models with errors that are close to the experimental errors, and those models can be very useful in saving time and expense.
In some cases, there is a clear need for computational science to "lead" experiments–to make predictions that can contribute to improving or falsifying a particular hypothesis. Computational and experimental science should be complementary, but in practice this does not often happen. Instead, we choose one method and abandon it only when it fails. Given the data, the choice of experimental science over computational science is a reasonable one.
Q: What should modelers know about crystallographic data that they currently do not?
A: Crystallographic data, like all other experimental data, contains measurement error and that error is measured. The inherent error affects the quality of the molecular model that is built to fit or explain the data. Crystallographic data and the resulting model do not have god-like properties such as infinite precision or perfect accuracy–just because a measurement is presented to three decimal places does not signify that the third decimal place has any meaning. The not-so-funny part is that modelers do understand model quality. We understand that a homology model built from a structure with 15% sequence identity is not as reliable as a model built from of a structure with 95% sequence identity. For some unexplained reason that understanding is not applied to crystallographic data, and too many believe that crystallographic models are infinitely precise.
Q: How has the interface between modeling and crystallography changed? What changes are likely to occur?
A: In the last 10 years, my perception has been that the interface has gone from one of two separate scientific silos launching data but rarely speaking to a more interactive team effort. Yes, there is still a silo effect because crystallography and modeling groups are only rarely collocated, but it is nothing like what it used to be. It is important to remember that we–in crystallography and modeling–each have customers. Crystallography has critically important data that modeling needs. Modeling uses the data to provide information to the drug discovery team. In all cases, the customer needs to understand the quality of the information being provided so that intelligent and scientifically sound decisions can be made. What changes would I like to see? I would like to see collocation of modeling and crystallography groups, and in the absence of that, consistent communication about the project being worked on and the quality of the data being generated. We need to remember that job security depends, in part, on demonstrated impact. We can have greater impact if we work together.
Q: How can crystallographic data best be used in modeling and
drug discovery?
A: The data can be best used if modelers and crystallographers each had a better understanding of each other’s field. That said, we do not all need to become fully rounded, Renaissance scientists, but a little understanding of the strengths and weaknesses of each discipline would allow the data to be used most effectively. If the crystallographer knows that the modeler will be using a technique that treats the protein as rigid but the data say that the protein is very flexible in the crystal, that information is potentially very important and may cause the modeler to use a different method. I have this unfounded belief that if a scientist understands the strengths and weakness of the data, they will use that information and do the right thing.
Q: What are the challenges that computational science and crystallography face with regard to drug discovery?
A: I would say the challenge is to have measurable impact. There are examples where crystallography and modeling have had an impact on the discovery and development of a marketed drug. Unfortunately, we need to have a consistent quantifiable effect on the drug discovery process; otherwise, from a business perspective we are not very useful. How can we have a consistent impact? By using the data being generated more efficiently. We need to understand the quality and accuracy of the data and use that information to generate predictions whose quality and accuracy are quantified. An understanding of how good or bad the data are, or a prediction is, allows us to determine when to use the data or seek other options. If crystallography and modeling can provide well-defined information, our impact will be obvious.
A Short Course: Crystallography for Modelers
Crystallography for Modelers addressed questions relating to the "quality" of the data used in modeling. How "good" are the protein structures being used? What's the error in atomic coordinates? How might you know if something is just plain wrong?
On May 7-8, 2009, practicing pharmaceutical and biophysical modelers attended the short course entitled Crystallography for Modelers to develop a better understanding of crystal structures and PDB data. The course was held at Rutgers, The State University of New Jersey in Piscataway, NJ and was sponsored by the RCSB PDB.
RCSB PDB members Helen Berman, Shuchismita Dutta, David S. Goodsell, and Cathy Lawson, along with Rutgers Professor of Chemistry and Chemical Biology Joseph Marcotrigiano, described the process of crystal structure determination and provided insight into the workings of the PDB and how "PDB" files are generated. In detailed discussions, participants examined the extensive information (beyond coordinates) available in data files and online, including ligand structures. Topics included proper interpretation of crystal structures, with an emphasis on accuracy, precision, problems and experimental, real, and/or perceived errors.
On the second day, industrial participants Jeffrey A. Bell (Schrödinger, Inc.), Gregory Warren (OpenEye Scientific Software, Inc.), Howard Feldman (Chemical Computing Group Inc.), and Ruben Abagyan (Molsoft LLC) led the class through hands-on software demonstrations.
Crystallography for Modelers was organized for the RCSB PDB by Terry Richard Stouch, and offered through the Rutgers Advanced Technology Extension program. The RCSB PDB gratefully acknowledges the additional support from Chemical Computing Group Inc., ACS Division of Computers in Chemistry, and the Journal of Computer-aided Molecular Design.
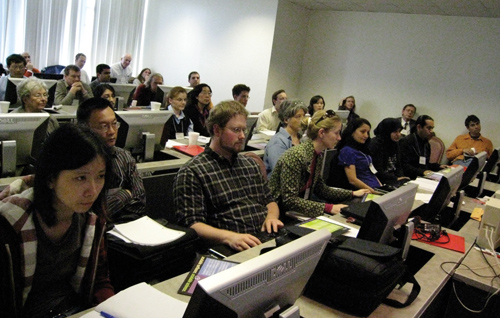
Course attendees were able to participate in hands-on activities. |